Agricultural Data Science
The Agricultural Data Science research group employs data-centric approaches to tackle agricultural carbon and nitrogen challenges.
Leveraging machine learning, meta-analysis, and process-based modelling, we improve the reliability in predicting carbon and nitrogen processes and evaluate the effectiveness of strategies to mitigate nitrogen pollution and greenhouse gas emissions in agroecosystems. We establish sustainable nitrogen indices, aiming to substantiate Australia’s ‘clean and green’ food reputation and guiding consumer choices while incentivising sustainable farming practices. In collaboration with the HyperSens Lab, we utilise remote sensing and GIS to analyse spatio-temporal data, enabling optimal resource allocation and precise decision-making in agricultural production.
News and events
Contact the team
Dr Alexis Pang
Lecturer, Precision Agriculture and Soil Science
Dr Shu Kee (Raymond) Lam
Senior Lecturer, Climate Change and Biogeochemistry
Group co-leaders
The Agricultural Data Science research group focuses on creating opportunities for innovation in big data to support the global efforts towards sustainable intensification of agriculture.
-
State-of-the-art instruments for trace gas measurements using micrometeorological techniques
Real-time, continuous field-scale measurement of greenhouse gas emissions from farms.
-
HyperSens Lab
The Hyperspectral and Thermal Remote Sensing Laboratory (HyperSens) is established jointly between the School of Agriculture, Food and Ecosystem Sciences (SAFES), Melbourne Veterinary School (MVS), and the Melbourne School of Engineering (MSE), Department of Infrastructure Engineering.
Our researchers and graduate research students.
Academic staff
A/Prof Shu Kee Lam
Shu Kee (Raymond) Lam's research focuses on soil carbon and nitrogen dynamics in agroecosystems, including soil-plant interactions under climate change (elevated atmospheric CO2 concentration) and mitigation of greenhouse gas emissions using urease and nitrification inhibitors. He also has expertise in global data synthesis (including meta-analysis).
shukee.lam@unimelb.edu.au +61390359619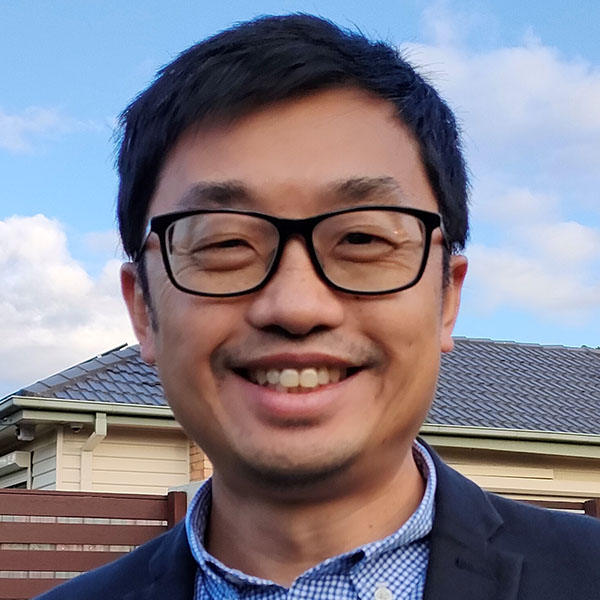
Dr Alexis Pang
Dr. Alexis Pang’s research interests rely in the area of precision agriculture, specifically the spatially and temporally variable water and nutrient management.
alexis.pang@unimelb.edu.au +61383447190Dr Baobao Pan
Baobao Pan's research focuses on soil carbon and nitrogen dynamics in agro-ecosystems by integrating big data analytics, machine learning techniques and process-based models.
baobao.pan@unimelb.edu.auDr Emma Liang
Xia (Emma) Liang’s research focuses on reactive nitrogen assessment, nitrogen footprint quantification, sustainable agriculture indicators and food credentialing at multiple scales, from local through to global levels. She also has expertise in global data synthesis and GIS. Her work is an exciting new research field nationally and internationally.
liang.xia@unimelb.edu.auGraduate researchers

Chuanzhen Zhang

Zhonghua Ma

Huirong Jing

Yifan Cao

Jingyi Xu
The Agriculture Data Science research group focuses on creating opportunities for innovation in big data to support the global efforts towards sustainable intensification of agriculture.
Modelling, machine learning and meta-analysis
To achieve efficient agricultural nitrogen (N) management, our group applies process-based agroecosystem simulation models to simulate, predict and evaluate the impacts of management practices on N losses through different pathways, thereby developing decision-support tools. More recently, we integrated agricultural big data with machine learning to revamp existing parameters of agroecosystem models and improve the reliability of model prediction in N processes. We have also applied meta-analytic techniques to national and international datasets on soil-plant carbon and N dynamics in agricultural systems and their mitigation and adaptation to climate change.
Sustainable nitrogen indices
Australia has a reputation globally for production of food that is ‘clean and green’, but this branding is neither meaningfully defined nor readily verified. To substantiate this claim scientifically, our group has ongoing research, integrating vast datasets and resource-use models, for building the world’s first evidence-based N indices for (Australian) agricultural products that are embedded with an environmental cost-benefit analysis. The indices will help consumers to choose food products with low environmental footprints, incentivise farmers to adopt more sustainable N management practices and benchmark Australian agricultural production against international practices. Our group has also developed anew indicator, reactive nitrogen spatial intensity (NrSI), to identify Nr emission hotspots, indicate the potential environmental impacts of Nr, and assist management recommendations.
Remote sensing, GIS and precision agriculture
Rapidly advancing sensor systems on ground, air and space-borne platforms delivering hyperspectral and thermal remote sensing imagery at high spatio-temporal resolutions provide massive volumes of data to be analysed in novel ways, unveiling detailed insights into crop performance and their variability across large areas. Simultaneously, modern agricultural production systems generate large volumes of spatially referenced information such as yield, grain quality, soil moisture and weather data. This represents rich opportunities to develop smart farm systems that will allow growers make increasingly precise decisions to maximize yield potential while optimizing water and nutrients. Our group is working on developing new techniques and algorithms using hyperspectral and thermal remote sensing technology to detect biotic and abiotic crop stress under drought, heat, frost and nutrient deficiencies before symptoms are visible.